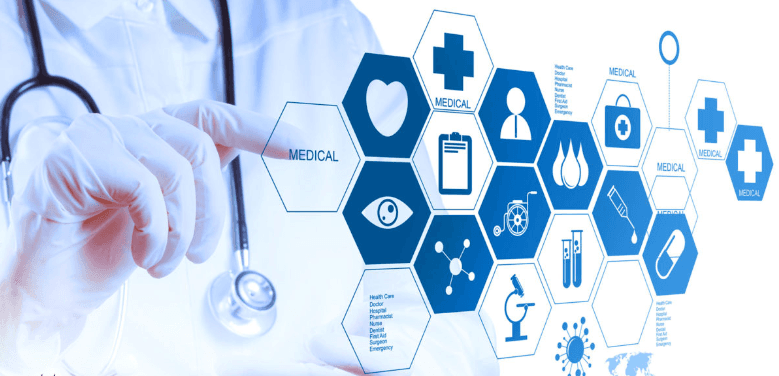
Cardiovascular Medicine
Machine learning is very helpful in solving problems, making it easy to do things. In the hospital or health sector, machine learning makes it easy to do something, for example a doctor can diagnose heart disease quickly without taking a long time. With the rapid increase in information about machine learning as a machine that can learn on its own without having to be controlled by each user, it has advantages and disadvantages.
Cardiovascular Medicine Machine
The advantage of this article is that everything is new, the article was published this year, and it provides details of the expected results and in short and clear writing. The disadvantage of this article is that the material or dataset used is relatively small and does not use a lot of data and uses references that are too long. Based on the results of research conducted, machine learning is very useful in the health sector and also in other fields, which makes everything easy.
Machine learning predicting the risk of hospitalization of HFpEF patients
An example of the recent application of machine learning in cardiovascular medicine is in predicting the risk of hospitalization of HFpEF patients with the reinforcement learning method. AI was used to classify 46 intrinsic patient variables based on demographic, clinical, and investigational data. Based on these data, AI created 3 phenotypic classifications that were used to predict mortality and hospitalization outcomes.
The results showed a significant increase in the risk of hospitalization by 4.2 times in group 3 compared to other groups, even after adjusting for risk factors. This AI-generated classification of HFpEF patients has even been successfully validated.
Deep Learning
The deep learning process resembles the human thought process by using multiple layers of artificial neuronal networks (ANN) to analyze nonlinearly and form automatic predictions from the given input data. Deep learning has great potential in image recognition for medical imaging. cardiovascular disease, as well as being able to “self-study” to find patterns from existing data (e.g. to find drug interactions). The disadvantage of deep learning is that it requires a large dataset so that it requires collaboration of various institutions and integrated electronic medical records, as well as the existence of a separate machine that is capable / has deep learning capacity.
Machine Learning
Machine learning, or machine learning, is the ability of computers to learn, which is generally grouped into 3 approaches: supervised, unsupervised, and reinforcement.
Supervised Learning
In supervised learning, the computer forms an algorithm according to the science/data that is inputted/labeled by humans to predict the outcome of a known patient dataset. The limitations of supervised learning are: it requires very large amounts of data so that it takes a long time because it must be manually labeled by humans, and requires repeated validation with other datasets to train the accuracy of the algorithm model.
Unsupervised Learning
In unsupervised learning, the computer forms an algorithm from the results of its own learning based on existing patient data. The advantage of unsupervised learning: can find hidden patterns from existing data to identify genotypes, phenotypes, and new disease mechanisms that have not been known by clinicians before. The disadvantage of unsupervised learning is that it is difficult to find initial patterns to form thinking algorithms (especially on noisy data such as echocardiographic images), so it requires manual coding in some ways at first, as well as repeated validation.
Reinforcement Learning
In reinforcement learning, computers use a combination of supervised and unsupervised learning, to maximize the accuracy of computer-generated yahoo from a trial and error process.
Machine learning functions
Machine learning functions are very diverse, in human daily life that cannot be separated from the development of an increasingly practical era, Machine learning is commonly used in various industries and fields, including:
Medical field
Machine learning can provide information related to a person's health during hospitalization. For example, an electrocardiogram machine that can detect heart disease early on.
It is conceivable that when each patient must be identified manually, this will certainly require a lot of doctors and personnel to oversee the monitoring process.
Machine Learning Applications in Cardiovascular Medicine
An example of the recent application of machine learning in cardiovascular medicine is in predicting the risk of hospitalization of HFpEF patients with the reinforcement learning method. AI was used to classify 46 intrinsic patient variables based on demographic, clinical, and investigational data. Based on these data, AI created 3 phenotypic classifications that were used to predict mortality and hospitalization outcomes.
The results showed a significant increase in the risk of hospitalization by 4.2 times in group 3 compared to other groups, even after adjusting for risk factors. This AI classification of HFpEF patients was even successfully validated in a prospective cohort of 107 patients. This shows the potential for using AI to stratify risk more easily and accurately than the current scoring system.
Deep Learning
The deep learning process resembles the human thought process by using multiple layers of artificial neuronal networks (ANN) to analyze nonlinearly and form automatic predictions from the given input data. Deep learning may be great at image recognition for medical imaging. cardiovascular disease, as well as being able to “self-study” to find patterns from existing data (e.g. to find drug interactions). The disadvantage of deep learning is that it requires a large dataset so that it requires collaboration between various institutions and integrated medical records, as well as the existence of a separate machine that is capable / has deep learning capacity.
Deep Learning Applications in Cardiovascular Medicine
Artificial intelligence with deep learning was found to be able to predict end-systolic and end-diastolic volumes through MRI, predict heart failure 9 months before the clinician's diagnosis was made, and analyze electrocardiographic (ECG) waves to be normal, abnormal, and threatening. soul with an accuracy rate of 99%.
Cognitive Computing
Cognitive computing is a way for computers to "self-learn" using machine learning or deep learning, as well as pattern recognition, to mimic human thought processes. It aims to create an algorithm model to solve problems without human assistance, for example IBM Watson. IBM Watson is a type of cognitive computing that "learns" continuously from various datasets (e.g. electronic medical) to predict various outcomes more accurately than humans.
Cognitive Computing Applications in Cardiovascular Medicine
The 2016 publication covered 50 patients using a cognitive computational artificial intelligence approach to differentiate constrictive pericarditis from restrictive cardiomyopathy using data on 15 variables from 3D-STE (3D speckle-tracking echocardiography) and 4 variables from echocardiography. The result is that the use of artificial intelligence can accurately diagnose constrictive pericarditis from restrictive cardiomyopathy, so that it is considered to be able to help interpret cardiac imaging, especially for novice medical personnel with limited experience.
The above study is still a pilot study with 50 patient samples so it requires further research, especially to compare it with the gold standard for the diagnosis of constrictive pericarditis. However, this study demonstrates the potential of artificial intelligence to help doctors use diagnosis more accurately.
Application of AI in Cardiovascular Sector in Indonesia
In Indonesia, which has a high prevalence of cardiovascular disease with limited cardiologists and health facilities, the use of AI can help better risk stratification. Health services in remote places can also be provided without the need for many specialists. However, there are limitations due to inadequate medical data, low follow-up rates and the lack of coordination in recording medical records within and between institutions.
Conclusion AI will play a bigger role in medicine, including in cardiovascular medicine. AI will help doctors to determine the diagnosis and treatment options that are tailored/customized for the patient. Examples of the role of artificial intelligence that continues to be developed in the cardiovascular field are to predict survival of HFpEF patients, predict end-systolic and end-diastolic volume via MRI, predict heart failure more quickly, analyze ECG waves, and differentiate constrictive pericarditis from restrictive cardiomyopathy. through echocardiography.